AI Hardware Requirements: A Comprehensive Guide
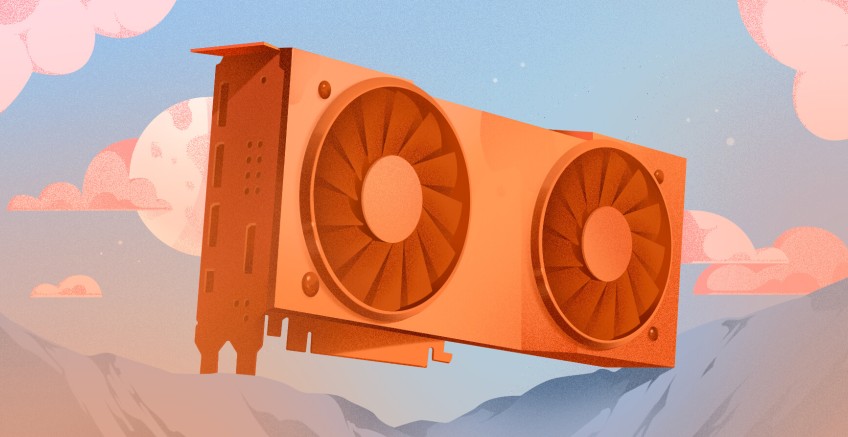
Deciding on your AI hardware setup can seem daunting, but a methodical process in selecting and configuring appropriate hardware can guarantee success. Understanding AI hardware requirements upfront can help avoid unnecessary costs while making scaling more efficient.
While many developers start their AI journey using platforms like Google Colab, Jupyter Notebooks, or Hugging Face, which manage computational demands via cloud services, individuals working on larger or more niche AI projects eventually reach the limits of consumer-level AI hardware. In this guide, I'll explain the exact AI hardware requirements for different workloads, listing each hardware component and comparing use cases.
#What is AI Hardware
AI hardware refers to the physical components and systems designed specifically to accelerate and optimize artificial intelligence workloads like machine learning (ML), deep learning, and neural network inference and training.
#AI Hardware Importance
The importance of hardware in AI projects cannot be overstated. When deploying AI systems on a larger scale, whether for research, development, or production, local hardware becomes critical. The right hardware will not only support complex machine learning models but also optimize processing speed, cost, and scalability.
AI models, particularly those using deep learning techniques such as convolutional neural networks (CNNs) or transformers, require significant computational power. The hardware utilized must be capable of managing huge datasets in addition to the parallel computations involved in both model training and inference.
#What hardware is needed for AI?
The AI hardware landscape has evolved significantly over the years. Initially, AI algorithms ran on CPUs (Central Processing Units), but as the complexity of models grew, GPUs (Graphics Processing Units) became the go-to hardware to speed up deep learning tasks.
📚Further reading: Best GPUs for Deep Learning
However, the choice of hardware—whether GPUs, CPUs, specialized accelerators like TPUs (Tensor Processing Units), or FPGAs (Field-Programmable Gate Arrays)—greatly impacts the overall performance of AI systems. Different hardware configurations are suited for different tasks, such as training large AI models or performing inference on trained models.
One important distinction in AI workflows is the difference between fine-tuning and inference, both of which have distinct hardware requirements.
Fine-tuning: involves training a model on domain-specific data, requiring significant computing power and memory. Inference: focuses on using an already-trained model to generate outputs, demanding less computational power but a higher focus on low-latency and cost-efficiency.
Rent Dedicated Servers Optimized for AI/ML Workloads
Dedicated bare metal cloud servers powered by new-gen AMD EPYC and Intel Gold CPUs and Nvidia GPU accelerators. Build your own custom AI server or deploy a pre-built server in minutes.
#AI Hardware Requirements
In AI, the AI hardware components you will require will be based on what you are doing. For instance, training a large neural network on a high-resolution dataset is not the same as executing small inference models in production. The first step is to understand the major components of your system.
- CPUs (Central Processing Units)
- GPUs (Graphics Processing Units)
- TPUs and FPGAs
- Cooling and Power Delivery
- Memory (RAM)
- Storage (NVMe SSDs)
Let's dive right in, and explore each in detail.
#1. CPUs: The Central Component for AI Workloads
At the center of every AI server is the CPU (Central Processing Unit). Although the CPU isn’t typically the powerhouse for deep learning tasks, it is responsible for data preprocessing, managing workloads, and communication across the various components. When choosing a CPU for AI tasks, look for the number of cores, clock speed, and cache size.
Option | Description |
---|---|
AMD Ryzen 7700X | 8-core processor with high clock speeds (~4.5 GHz). Excellent for AI workloads that involve parallel data handling and fast inference cycles. |
Intel Xeon Gold 6230R | Enterprise-grade CPU with 20 cores and high thread count. Ideal for large-scale AI workloads requiring heavy multitasking and sustained compute. |
The AMD Ryzen 7700X or Intel Xeon Gold 6230R are excellent options depending on your workload scale and budget. CPUs such as Intel Xeon or AMD EPYC processors are commonly used in AI infrastructures due to their high core count and excellent multi-threading capabilities.
A high core count allows for the parallel handling of multiple tasks, such as data loading and model management. Meanwhile, the clock speed impacts how quickly these tasks can be processed. Cache size is another crucial factor, as it provides faster access to frequently used data, minimizing delays in AI model training or data processing.
For AI training, especially when working with large datasets, it is essential to strike a balance between the number of cores and the clock speed. While more cores allow for parallelization, higher clock speeds can significantly improve tasks that require single-threaded operations.
#2. GPUs: Accelerating Deep Learning
While CPUs are essential for running AI systems, Graphics Processing Units (GPUs) are the real workhorses for training complex deep learning models. GPUs are highly efficient in executing parallel processing required by deep learning algorithms, making them ideal for training models like ResNet, GPT, and other neural networks.
Tier | Recommended GPU(s) | Use Case |
---|---|---|
Entry to Mid-Level | NVIDIA A2, NVIDIA A10 | Balanced performance and energy efficiency for training and inference on smaller to medium-scale models. |
Advanced | NVIDIA A40 | High memory bandwidth and DL optimizations for complex models and larger datasets. |
Enterprise-Scale | NVIDIA A100 | Premium performance for training large models (e.g., LLMs, deep vision), with up to 80 GB HBM2 memory. |
📚Further reading: Best GPUs for Machine Learning
The key specifications to look for when selecting a GPU for AI workloads are GPU cores, memory size (VRAM), and memory bandwidth. More GPU cores allow the GPU to handle more operations simultaneously, while large VRAM ensures that large models and datasets can be processed without performance bottlenecks. High memory bandwidth enables faster data transfer between the memory and processing cores, which is essential when working with high-resolution data or large neural networks.
For large-scale AI tasks, such as training models for natural language processing or computer vision, you’ll want to consider high-end GPUs like the NVIDIA A100 or RTX 4090. For smaller, more budget-conscious setups, NVIDIA RTX 6000 Ada or AMD’s Radeon Pro series can still offer significant performance while being more cost-effective.
#3. TPUs and FPGAs: Specialized Hardware for AI
In addition to GPUs, there are specialized accelerators such as Tensor Processing Units (TPUs) and Field-Programmable Gate Arrays (FPGAs) that can further optimize AI performance.
-
TPUs, designed by Google, are custom-built for tensor operations commonly used in deep learning, making them ideal for tasks like training large neural networks. They can provide significant speedups over traditional GPUs for certain types of models.
-
FPGAs are reconfigurable, allowing users to customize hardware for specific tasks, improving energy efficiency and performance for certain AI workloads. These are especially useful in environments where latency is critical, such as in real-time AI inference.
These specialized processors are typically used in large-scale data centers or by companies that need to push the boundaries of AI performance. They are powerful but need a greater level of experience to be integrated into your environment.
#Cooling and Power Delivery
High-performance AI servers, particularly those equipped with GPUs, TPUs, and multiple processing units, generate substantial heat due to the intensive parallel computations required during training and inference tasks. Efficient thermal management is essential to prevent thermal throttling, which can degrade performance and shorten hardware lifespan. Advanced cooling systems, including liquid cooling or direct-to-chip cooling solutions, are often deployed to ensure stable operating temperatures by efficiently dissipating heat.
Moreover, stable and redundant power delivery is critical for maintaining uninterrupted performance. AI servers require high-wattage power supply units (PSUs) capable of providing consistent power to all components, including GPUs, CPUs, and memory. Using modular PSUs with high-efficiency ratings (80 Plus Platinum or Titanium) ensures minimal energy loss and stable voltage regulation, which is crucial for preventing power-related bottlenecks during peak load conditions.
#4. Memory: How Much RAM for AI?
Balancing size and speed is important. AI workloads involve processing massive amounts of data, which requires not only powerful CPUs and GPUs but also ample memory and fast storage solutions.
Workload Type | Recommended RAM |
---|---|
Smaller Workloads | Minimum: 32 GB |
Larger Models & Datasets | Recommended: 64–128 GB |
Having sufficient RAM is crucial when working with large datasets, as it allows the system to quickly access and process data without relying on slower storage. For AI tasks, it’s recommended to have at least as much RAM as the GPU’s VRAM. This ensures that the system can hold large datasets in memory while the GPU performs intensive computations.
For projects involving large datasets or high-resolution data, opting for 128GB or more RAM will help avoid slowdowns caused by swapping data between memory and storage. Additionally, ECC (Error-Correcting Code) RAM can be a crucial addition in mission-critical AI projects where data integrity is essential, especially during long-running computations.
#5. Storage: Speed and Scalability
AI systems also require high-speed storage solutions. NVMe SSDs offer the best performance in terms of data access speeds, ensuring that datasets can be loaded into memory quickly. These drives are particularly important for AI applications where fast data throughput is essential, such as real-time processing or training on large datasets.
Setup Size | Recommended Storage |
---|---|
Standard Workloads | 500 GB NVMe SSD |
Heavy Workloads / Datasets | 1 TB+ NVMe SSD |
For cold storage (i.e., data that isn’t frequently accessed but still needs to be stored), SATA SSDs or traditional HDDs can provide more affordable options for archiving. The ideal solution involves using NVMe SSDs for active data processing and larger storage devices like HDDs for long-term data storage.
For enhanced performance and redundancy, consider RAID 0 (speed) or RAID 1 (mirroring) configurations—especially in production environments.
#Fine-Tuning vs. Inference: Hardware Needs for Each Stage
The hardware requirements for fine-tuning AI models differ significantly from those required for inference.
1. Fine-tuning
Fine-tuning involves training a model on specific data with the goal of adapting it to a new task. This stage requires significant computing power, typically relying on high-end GPUs or TPUs with ample memory and high-speed networking. Fine-tuning can be an intensive and time-consuming process, especially when training on large datasets or deep models.
2. Inference
Inference is the stage where a trained model is used to generate predictions based on new input data. While inference doesn't require as much computational power as fine-tuning, it demands low latency and high throughput to ensure that predictions are made in real-time or near real-time. Smaller GPUs, like the NVIDIA T4 or A10, are often preferred for inference tasks, as they provide a balance between performance and cost. CPUs may also be sufficient for smaller models or when low latency is not a priority.
#Additional Considerations: Networking
Networking powers distributed AI systems. As AI applications scale, many models are trained across multiple servers in a distributed setup, requiring fast and efficient data transfer between nodes. Low-latency, high-bandwidth networks, like InfiniBand or 100GbE Ethernet, are crucial to ensure quick communication and avoid bottlenecks.
Cloud-based services such as AWS, Azure, and Google Cloud offer specialized AI instances, providing high-end GPUs and networking resources without the need for on-premises hardware. For more flexible and cost-efficient solutions, SMEs like Cherry Servers offer scalable cloud infrastructure optimized for AI workloads, giving businesses access to powerful GPUs, low-latency networking, and optimized storage.
#Conclusion
The choice of hardware for AI projects—whether for fine-tuning, training, or inference—depends on the specific demands of your workload. While powerful GPUs and TPUs are essential for training deep learning models, CPUs, RAM, and storage solutions play key roles in ensuring that your system is well-equipped for data processing and model management. Understanding the hardware needs of each phase in the AI pipeline is crucial to building a system that maximizes efficiency while minimizing costs.
Dedicated Servers Optimized for AI/ML Workloads
Deploy your application on dedicated hardware designed to meet AI requirements.