Deep Learning Hardware: Requirements and Setup
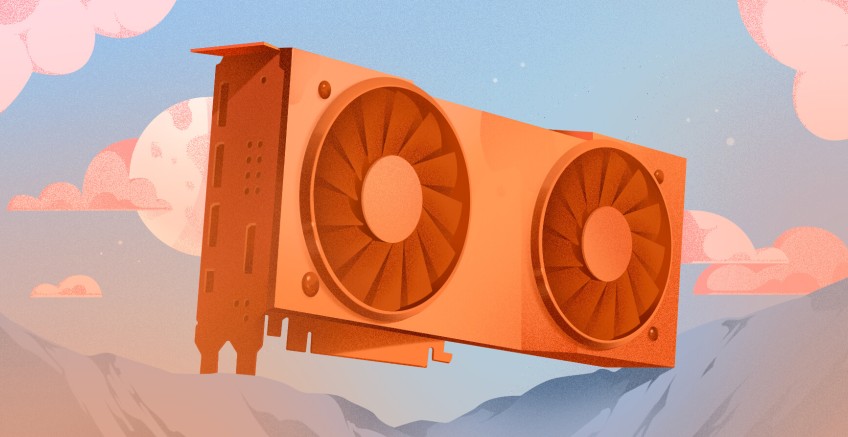
Deep learning has become the cornerstone of modern artificial intelligence, powering everything from autonomous vehicles to real-time language translation. The large-scale models used for these applications rely on immense amounts of data and computational resources. However, the hardware traditionally used for computing, such as general-purpose Central Processing Units (CPUs), often struggles to keep up with these growing demands.
As a result, specialized hardware accelerators such as Graphics Processing Units (GPUs), Tensor Processing Units (TPUs) and Field Programmable Gate Arrays (FPGAs) have become essential for efficiently training and deploying deep learning models. In this guide, we’ll explain the different types of deep learning hardware, practical considerations when choosing hardware for your project, and how to integrate these components effectively into your workflow.
#What is Deep Learning Hardware?
Deep learning models are highly complex, consisting of layers of interconnected neurons that process and analyze vast datasets. These models require significant computational power, not only to train but also to infer from large datasets in real-time. The complexity of these models leads to massive parameter spaces and high data throughput demands. As traditional CPUs are not optimized for such parallel tasks, specialized hardware accelerators offer superior performance by processing many calculations simultaneously.
-
GPUs and TPUs: These accelerators, unlike CPUs, are optimized for parallel computation. GPUs handle large-scale matrix operations and tensor computations effectively, while TPUs are purpose-built by Google to handle high-throughput tensor operations used in deep learning.
-
Why specialized hardware is needed: The size and complexity of deep learning models require hardware that can deliver massive parallelization to avoid bottlenecks during training. The result is faster model training and inference times, which makes specialized hardware essential for deep learning.
#Deep Learning Hardware Architecture and Requirements
Building a deep learning system requires careful selection of components to support the intensive computational needs of modern AI models. We’ll go over these briefly. Below, you can find a general overview of deep learning hardware architecture.
#Central Processing Units(CPU)
At the heart of the system is the CPU, responsible for managing general tasks and enabling high-speed data preprocessing through multi-threading.
Workload Type | Minimum Specs | Recommended Specs |
---|---|---|
Demanding Workloads | 16-core / 32-thread (e.g., AMD Ryzen Threadripper 5955WX, Intel Xeon W-3345) | 32-core+ (e.g., AMD Threadripper PRO 7995WX) |
Less Demanding Workloads | 8-core / 16-thread (e.g., AMD Ryzen 7700X) | 12-core / 24-thread (e.g., Core i7-14700K) |
#Graphics Processing Units(GPU)
The GPU serves as the primary workhorse, offering massive parallelization essential for model training, with high VRAM crucial for handling large datasets efficiently. For TensorFlow-specific workloads, TPUs (Tensor Processing Units) offer specialized acceleration.
Workload Type | Minimum Specs | Recommended Specs |
---|---|---|
Demanding Workloads | NVIDIA RTX 4090 (24 GB) / NVIDIA A100 (40–80 GB VRAM for enterprise) | H100 or multiple A100s for cutting-edge training |
Less Demanding Workloads | NVIDIA RTX 4070 (12 GB) / RTX 4080 (16 GB) | NVIDIA L4 GPU for efficient AI inference |
#Random Access Memory (RAM)
Sufficient memory (RAM) is needed to handle extensive datasets, while system RAM supports data preprocessing, and GPU VRAM holds model parameters during training.
Workload Type | Minimum Specs | Recommended Specs |
---|---|---|
Demanding Workloads | 128 GB DDR5 RAM | 256 GB or more (for very large datasets or concurrent multi-user training sessions) |
Less Demanding Workloads | 32 GB DDR5 RAM | 64 GB RAM for larger batch sizes |
#Storage
Fast storage, preferably NVMe SSDs, ensures low-latency access to datasets and checkpoints, while RAID setups provide redundancy and enhanced throughput for mission-critical environments.
Workload Type | Primary Storage | Secondary Storage | Optional |
---|---|---|---|
Demanding Workloads | 2 TB NVMe Gen 4 SSD | 4–8 TB SATA SSD / Enterprise HDD | RAID 0 (speed) / RAID 1 (redundancy) |
Less Demanding Workloads | 1 TB NVMe SSD | 2–4 TB HDD | – |
#Power Supply (PSU)
A compatible motherboard must offer adequate PCIe slots to support multiple GPUs, and a robust power supply (PSU) is essential for stable performance under heavy loads.
Workload Type | Minimum Specs | Recommended Specs |
---|---|---|
Demanding Workloads | 1200W Platinum-rated PSU | 1600W Platinum PSU for multi-GPU |
Less Demanding Workloads | 750W Gold-rated PSU | 850W PSU for upgrade flexibility |
#Cooling
As deep learning tasks can generate significant heat, effective cooling solutions are critical, especially in multi-GPU systems, to avoid thermal throttling.
Workload Type | Recommended Cooling Solutions |
---|---|
Demanding Workloads | Custom liquid cooling or top-tier air cooling solutions (e.g., Noctua NH-D15) with enhanced airflow |
Less Demanding Workloads | High-end air cooling, with temperature monitoring |
#Private Cloud/Hybrid Options
For scaling beyond local setups, rack-mounted servers and cloud solutions like AWS or Google Cloud provide flexibility and scalability. However, bare metal private cloud providers like Cherry Servers offer the control and security of on-premises infrastructure while incorporating flexibility and scalability of public clouds, making it a versatile solution for organizations with specific needs.
Usage Type | Server Specs | GPU Options |
---|---|---|
Light to Medium Workloads | AMD EPYC 7402P (24 cores, up to 512 GB RAM) | NVIDIA A10 (24 GB) or NVIDIA A2 GPUs |
Heavy Workloads | Dual AMD EPYC 7443 (48 cores, up to 1024 GB RAM) | NVIDIA A40 (48 GB) / NVIDIA A100 (80 GB) |
Lightweight Inference / Hosting | Intel Gold 6230R (26 cores, up to 384 GB RAM) | Tesla P4 (8 GB) / Quadro K2200/K4200 |
#How to Choose the Best Hardware for Deep Learning
Selecting the best hardware for your deep learning project is critical for optimizing performance and ensuring that your system can handle the computational demands of large AI/ML models. Your choice of hardware will largely depend on the size of the models you're working with, the nature of the tasks you're performing, and your project's budget. Below is a detailed breakdown of factors you should consider when choosing the right hardware.
Rent Dedicated Servers Optimized for AI/ML Workloads
Dedicated bare metal cloud servers powered by new-gen AMD EPYC and Intel Gold CPUs and Nvidia GPU accelerators. Build your own custom AI server or deploy a pre-built server in minutes.
#Model Size and Complexity
The complexity and size of your deep learning models are perhaps the most important factors in determining the hardware you’ll need. Larger models with more parameters require hardware that can process massive amounts of data simultaneously.
For example, if you are working on complex tasks like natural language processing (NLP) (such as training models like GPT-3) or image recognition (using deep neural networks), GPUs are typically the best choice. They excel in performing parallel computations, enabling them to handle the complex matrix operations required for these models.
For smaller models or simpler tasks like classification of small datasets or basic regression models, you might be able to use a CPU. CPUs are perfectly adequate for inference tasks (applying a trained model to make predictions), which typically don’t require the high levels of parallel computation needed during the training phase.
#Batch Size and Training Iterations
The batch size (the number of data samples processed in one training iteration) directly impacts the hardware requirements. Large batch sizes require a lot of memory, especially VRAM (Video RAM) in GPUs. For instance, training a model on a dataset like ImageNet might involve a batch size of 256 or more, demanding GPUs with large memory capacities (e.g., 24GB VRAM on RTX 3090).
Smaller batch sizes can often be managed with mid-range GPUs or even CPUs if your model isn’t too complex. As your batch size increases, however, so does the need for greater memory bandwidth and GPU processing power, making high-end GPUs or specialized TPUs (Tensor Processing Units) essential for maintaining performance.
#Training vs. Inference Hardware
When deciding on hardware, it's important to differentiate between the hardware needed for training and inference. Training a model requires significantly more computational power because it involves continuously adjusting the model’s parameters over many iterations (epochs). GPUs and TPUs are ideal for this task due to their ability to perform parallel calculations across multiple cores.
In contrast, inference (using a trained model to make predictions) doesn’t require the same level of computational resources. While CPUs can handle most inference tasks, using a GPU or TPU can still provide a significant speedup, especially when handling large volumes of inference data or making real-time predictions.
For example, TensorFlow models run significantly faster on TPUs, particularly in cloud environments where hardware scaling can be done efficiently. However, if the model is already trained and only requires inference on new data, a CPU or a mid-tier GPU like the RTX 3060 can suffice for smaller projects.
#Cloud vs. On-Premise Solutions
Choosing between cloud-based or on-premise hardware largely depends on your project's scale, budget, and long-term needs.
-
Cloud Hosting: Cloud platforms like AWS, Google Cloud, and Microsoft Azure provide flexible, scalable solutions for deep learning projects. You can access high-performance hardware like GPUs, TPUs, and FPGAs without the need for large upfront investments in hardware. Cloud services allow you to scale your resources based on demand, which is ideal for projects with fluctuating resource needs or limited budgets. For instance, Google Cloud's TPU v3 is highly optimized for TensorFlow workloads, allowing for faster training of large models without investing in on-premise hardware.
-
On-Premise Hardware: On-premise setups offer more control over your hardware and can be more cost-effective in the long run if you have ongoing, high-performance workloads. For example, organizations with large-scale training jobs that need dedicated resources might opt for servers with multiple NVIDIA A100 GPUs.
On-premise setups can also be beneficial for data security and latency-sensitive models, where hardware location is critical. However, they do come with higher upfront costs and the need for regular maintenance.
#Types of Deep Learning Hardware
When selecting hardware, you have a few choices depending on the nature of your project:
-
CPUs are generally for smaller models or inference tasks, where parallel processing is less crucial. For heavier training, however, GPUs or TPUs are far more effective.
-
GPUs, especially those from NVIDIA, are the preferred choice for most deep learning tasks. Cards like the A100, V100, and RTX 3090 offer high performance and are optimized for parallel tasks, reducing training time significantly.
-
TPUs provide exceptional performance for models built in TensorFlow and are ideal for matrix-heavy operations.
-
FPGAs offer specialized acceleration for certain types of deep learning models. While they require more setup and expertise, they are highly efficient for low-latency tasks.
#Key Considerations in Deep Learning Hardware
When selecting hardware for deep learning, it’s crucial to consider factors like computational power, memory, scalability, and storage to ensure smooth operation throughout your project.
Computational Power and Scalability: For deep learning, parallel processing is essential. GPUs excel in this area, providing massive parallelization across hundreds or thousands of cores. As your dataset and model size grow, the ability to scale across multiple machines or nodes becomes important. This is especially true for large-scale projects that require distributing training across a cluster of GPUs.
Memory and Storage: Both system RAM and GPU VRAM are critical for handling large datasets and model parameters. Insufficient memory can lead to crashes or slow training times. As model complexity increases, so do memory requirements. SSDs are preferred over HDDs for storing datasets due to their faster read/write speeds, which reduces bottlenecks during training.
Power and Cooling: Deep learning hardware consumes significant power, particularly GPUs and TPUs. Efficient power management and cooling are essential to maintain performance. Liquid cooling can be an effective solution for multi-GPU systems, ensuring stable operation during long training runs.
#Conclusion
Selecting appropriate hardware for your deep learning project is imperative in achieving optimal performance and scalability. From CPUs to GPUs, TPUs, and FPGAs, each type of hardware has its advantages based on the specific task you are faced with.
Regardless of whether you decide to put your hardware in the cloud or on-premises, the most important factor is aligning the hardware with the specific requirements of your project.
Cherry Servers offer customizable AI/ML hosting optimized for deep learning applications, with ready-to-deploy configurations based on latest-generation AMD EPYC and Intel Gold CPUs, and optional NVIDIA accelerators like the A10, A40, and A100 GPUs.
Dedicated Servers Optimized for AI/ML Workloads
Deploy your application on dedicated hardware designed to meet AI requirements.